Being right is not enough
The challenge of epistemology
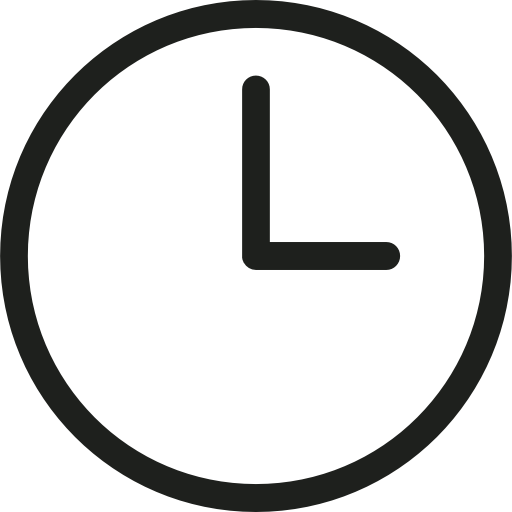
“It’s Difficult to Make Predictions, Especially About the Future” — Yogi Berra
A thief goes through the window in the middle of the night to make a grand jewelry heist. While tiptoeing inside the apartment, he sees the longcase clock with the time 1.05. He knows that the owners will be back at 1.15 AM. He decides that the safest thing for him is to exit and escape without getting the precious necklace. He can try again later. Unbeknownst to him, the clock had stopped working 12 hours ago, but at that moment, it just happened to be 1.05 AM.
After the American philosopher Edmund Gettier, our thief experienced what has been dubbed a Gettier problem. These problems’ archetypes challenge the classical criteria for knowledge: justification, truth, and belief. In our example, the thief is justified in believing it’s 1.05 AM, and it is 1.05 AM, and he believes it. Yet he doesn’t have knowledge because the instrument he relies on is faulty.
I recently had an online conversation with someone named Bob. Bob thought that Nate Silver’s forecast that Donald Trump had one-third of a chance of winning the American presidency in 2016 was a good ex-ante prediction. His justification was that no one thought he had that high of a chance and that, in the end, he won. Yet the obvious flaw in this reasoning is that an unbiased coin could have done better.
Imagine an alternate prognosticator in 2016: Jade Gold. Jade decides to predict the outcome of the 2016 presidential election by using a gold coin. Heads, it’s going to be Trump, and tails, it’s going to be Hilary. After a hundred tosses, she claims, “Trump has a 50 percent chance of winning the American presidential election”. After being hailed as a super prognosticator following Trump’s election by my internet friend, would her revealing that she used a coin to predict the election have an effect?
Would Nate silver’s statistical analysis of polls and social media hold more weight than Jade’s prediction when he was worse than a coin flip?
Philosophy, as they say, helps you think critically. Still, when atmospheres are charged, and issues are politicized, even the best explanation of facts cannot help you get your point across.
Years ago, as I was cutting my teeth as an architect, I was asked to evaluate two databases on non-functional requirements to select the best among them. I googled the term “Non-Functional Requirements” and came up with several criteria for the operations of the database. I didn’t know at the time and didn’t bother to google the deeper meaning of ilities (which I will expand on later).
I was tasked with working on those criteria with another architect from the client named Lee. Apparently, Lee didn’t know what non-functional requirements were, but he was acquainted with English vocabulary and elementary logic. We met with his manager, who was not very technical, and Lee had something to prove. In the meeting, I told them that NFRs meant a database’s performance characteristics, such as: How long it takes to perform a read and a write and what are the odds of losing data. I even showed them the article. The young Lee retorted to me, “Well if these are non-functional requirements, what are the functional requirements.” Not knowing the full history of the term, I couldn’t reply. So, he created a grid with such exotic criteria as “Number of stars on GitHub” and “how big is the open-source community that support the two databases.” All is well, but these were not NFRs.
NFRs— whose nomenclature some refer to as a misnomer — are named as such to be distinguished from software business requirements. For a database, the functional requirements would be creating the various schemas and saving the various data fields in the database. A better term for NFRs is ilities to refer to reliability, availability, scalability, usability, and maintainability. But the term NFR stuck, and in that meeting, it didn’t do my client or me any good.
Being right is not enough. You must get your message across to the other side to accomplish anything.
Going back to Bob, he distinguished between ex-ante predictions and ex-post results. These terms refer to “before the event” and “after the fact” in Latin. The idea that Nate Silver’s was a good prediction ex-ante comes from the notion that most people in the media at the time were predicting a Clinton landslide. After the fact, Nate Silver’s one-third chance of a Trump victory looks high, ex-post. Yet the problem with this type of reasoning is that it is highly subjective. The classic example for applying ex-ante and ex-post reasoning is probabilistically measurable data, such as a lottery. You know from the rules of how lotteries are drawn that ex-ante you are likely to lose, and for a lucky person ex-post, they will win. But in a presidential election forecast, what matters are the above 50% predictions ex-ante that pan out to be true ex-post.
At the same time, we will not give any credence to Jade Gold’s prediction of a 50% Trump victory, just like we don’t consider Gettier’s cases knowledge. We care about how we arrive at knowledge as much as the knowledge itself. This brings me back to the NFR case. Realizing that my interlocutor had an agenda and that I didn’t know the full history of the term, we should have taken a break and dug deeper later. As it happened, one of my colleagues had a good explanation for what NFRs were. As for Bob, years of arguing online have taught me one important lesson, you can’t win an online argument. You just let your likes do the winning for you!
Comments
Post a Comment